UAV & Drones
This project focuses on solving various issues related to networking in UAV's including security, charge scheduling etc.
Team Members
- Vinay Chamola (Senior Member, IEEE)
- Vikas Hassija
- Tejasvi Alladi
Collaborators
- Mohsen Guizani, Qatar University (Fellow, IEEE)
- Dusit Niyato, Nanyang Technological University, Singapore (Fellow, IEEE)
- Richard Yu, Carleton University, Canada (Fellow, IEEE)
Our publications in UAV and drones
Abstract
Use of Unmanned Aerial Vehicles (UAVs) is rapidly increasing in various domains such as disaster management, delivery of goods, surveillance, military, etc. Significant issues in the expansion of UAV-based applications are the security of (IoT to UAV) communication, and the limited flight time of the UAVs and IoT devices considering the limited battery power. Standalone UAVs are not capable of accomplishing several tasks, and therefore swarm of UAVs is being explored. Security issues in the swarm of UAVs do not allow the applications to leverage the full benefits that one can offer. Several recent studies have proposed the use of a distributed network of UAVs to upgrade the level of security in the swarm of UAVs. In this paper, a framework for secure and reliable energy trading among UAVs and charging stations is presented. Advanced blockchain, based on the tangle data structure is used to create a distributed network of UAVs and charging stations. The proposed model allows the UAVs to buy energy from the charging station in exchange for tokens. If the UAV does not have sufficient tokens to buy the energy, then the model allows the UAV to borrow tokens from the charging station. The borrowed tokens can be repaid back to the charging station with interest or late fees. A game-theoretic model is used for deciding the buying strategy of energy for UAVs. Numerical analysis shows that the proposed model helps in providing increased utility for the swarm of UAVs and charging stations in a secure and cost-optimal way as compared to the conventional schemes. The results can eventually be applied to IoT devices that constantly need energy to perform under ideal conditions.
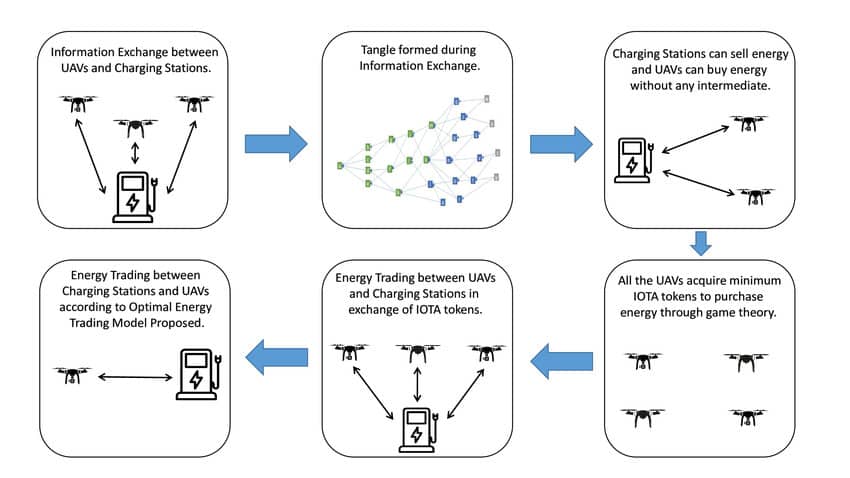
Abstract
The unprecedented outbreak of the 2019 novel coronavirus, termed as COVID-19 by the World Health Organization (WHO), has placed numerous governments around the world in a precarious position. The impact of the COVID-19 outbreak, earlier witnessed by the citizens of China alone, has now become a matter of grave concern for virtually every country in the world. The scarcity of resources to endure the COVID-19 outbreak combined with the fear of overburdened healthcare systems has forced a majority of these countries into a state of partial or complete lockdown. The number of laboratory-confirmed coronavirus cases has been increasing at an alarming rate throughout the world, with reportedly more than 3 million confirmed cases as of 30 April 2020. Adding to these woes, numerous false reports, misinformation, and unsolicited fears in regards to coronavirus, are being circulated regularly since the outbreak of the COVID-19. In response to such acts, we draw on various reliable sources to present a detailed review of all the major aspects associated with the COVID-19 pandemic. In addition to the direct health implications associated with the outbreak of COVID-19, this study highlights its impact on the global economy. In drawing things to a close, we explore the use of technologies such as the Internet of Things (IoT), Unmanned Aerial Vehicles (UAVs), blockchain, Artificial Intelligence (AI), and 5G, among others, to help mitigate the impact of COVID-19 outbreak.
Abstract
The recent advancement in Unmanned Aerial Vehicles (UAVs) in terms of manufacturing processes, and communication and networking technology has led to a rise in their usage in civilian and commercial applications. The regulations of the Federal Aviation Administration (FAA) in the US had earlier limited the usage of UAVs to military applications. However more recently, the FAA has outlined new enforcement that will also expand the usage of UAVs in civilian and commercial applications. Due to being deployed in open atmosphere, UAVs are vulnerable to being lost, destroyed or physically hijacked. With the UAV technology becoming ubiquitous, various issues in UAV networks such as intra-UAV communication, UAV security, air data security, data storage and management, etc. need to be addressed. Blockchain being a distributed ledger protects the shared data using cryptography techniques such as hash functions and public key encryption. It can also be used for assuring the truthfulness of the information stored and for improving the security and transparency of the UAVs. In this paper, we review various applications of blockchain in UAV networks such as network security, decentralized storage, inventory management, surveillance, etc., and discuss some broader perspectives in this regard. We also discuss various challenges to be addressed in the integration of blockchain and UAVs and suggest some future research directions. Index Terms-Unmanned Aerial Vehicle (UAV) network, security and privacy, blockchain technology, Internet of Things (IoT).
Abstract
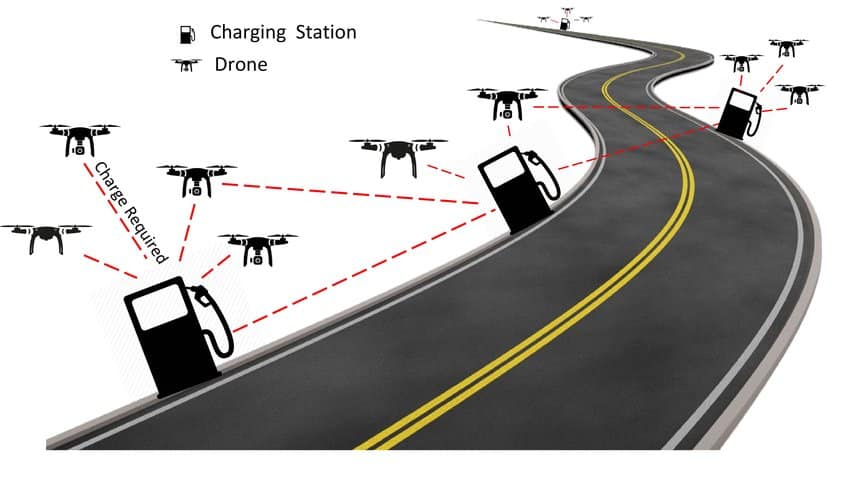
Abstract
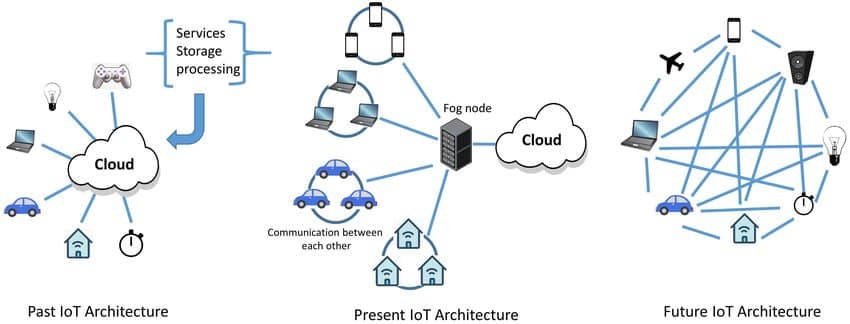
Abstract
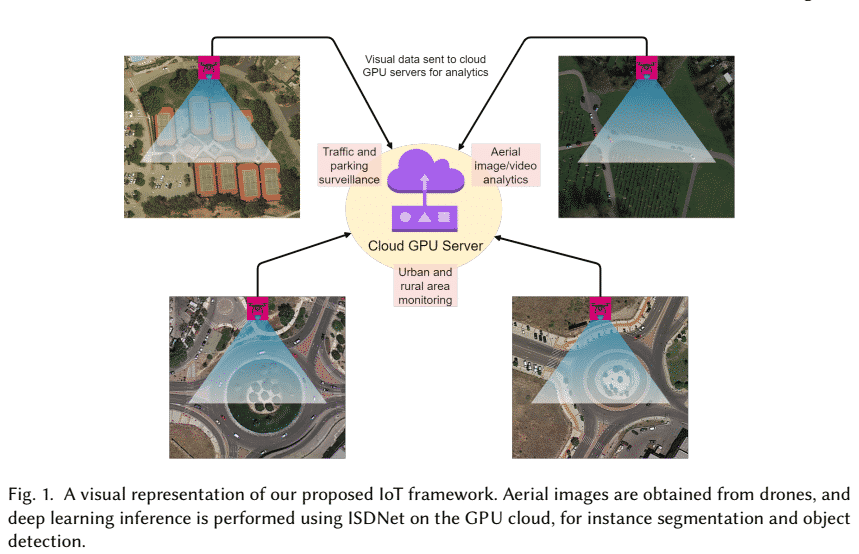