Healthcare Technologies
Team Members
- Vinay Chamola (Senior Member, IEEE)
Collaborators
- K.K. Raymond Choo, UTSA, USA
Our publications in Healthcare Technologies
Abstract
Abstract
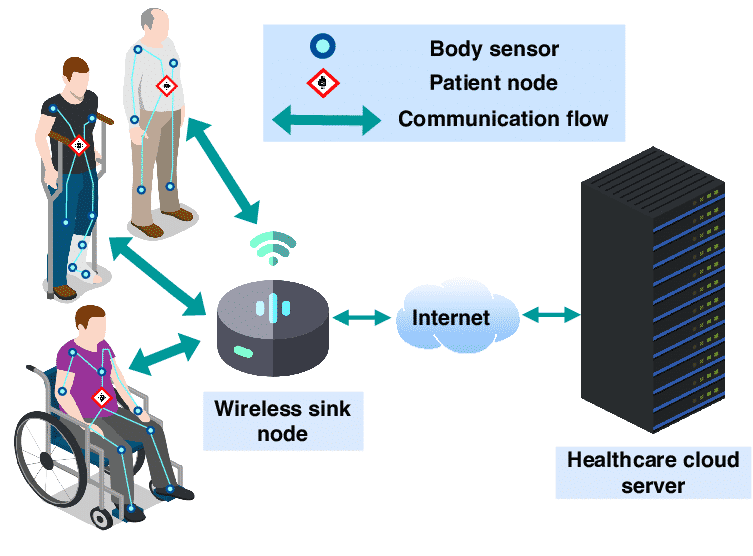
Abstract
Abstract
Abstract
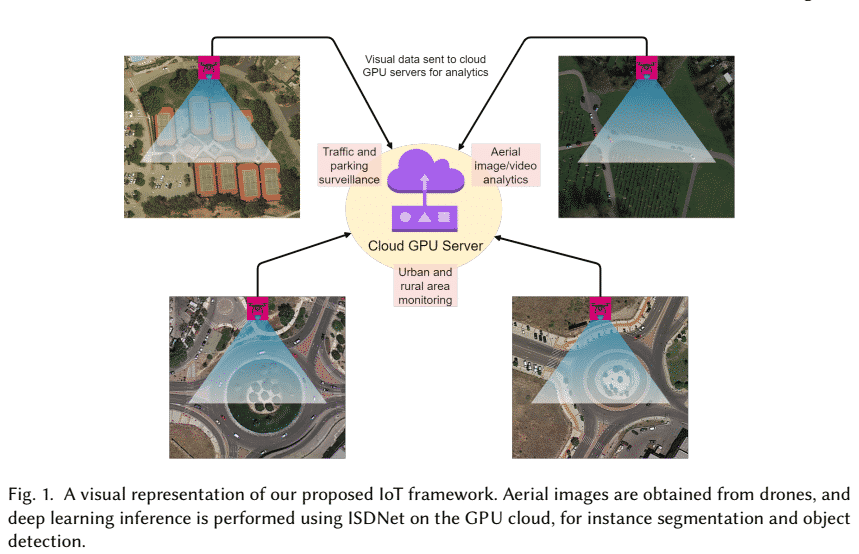
Abstract
Parking lot allocation problem has received much attention in recent years. There have been various works in the literature that target the parking slot allocation problem. However, most of these works use algorithms that run on centralized servers and are based on some predictions on historical data. Due to the dynamic nature of vehicular networks, the accuracy of such prediction models is not high which ends up in a chaotic situation for the parking lot owners as well as the vehicle owners. Therefore, a distributed Parking slot Allocation Framework based on Adaptive Pricing Algorithm and Virtual Voting is proposed in this paper. The proposed model is based on virtual voting and hashgraph consensus algorithm. Using the model, all users and parking lot owners can easily come to consensus finality about the allocation of a parking slot with the use of minimal bandwidth. The proposed model provides a fair, fast and cost-optimal parking slot allocation method. The perfect ordering of allocation requests is also maintained based on consensus timestamp. Further, an adaptive pricing model is proposed to enhance the overall revenue of the parking lot owners and comfort of the users. The proposed model is deterministic and can reduce the average parking cost and time. Performance evaluations reveal that the proposed model outperforms its counterparts in terms of accurate parking slot allocation, reduced cost and parking lot resource utilization.
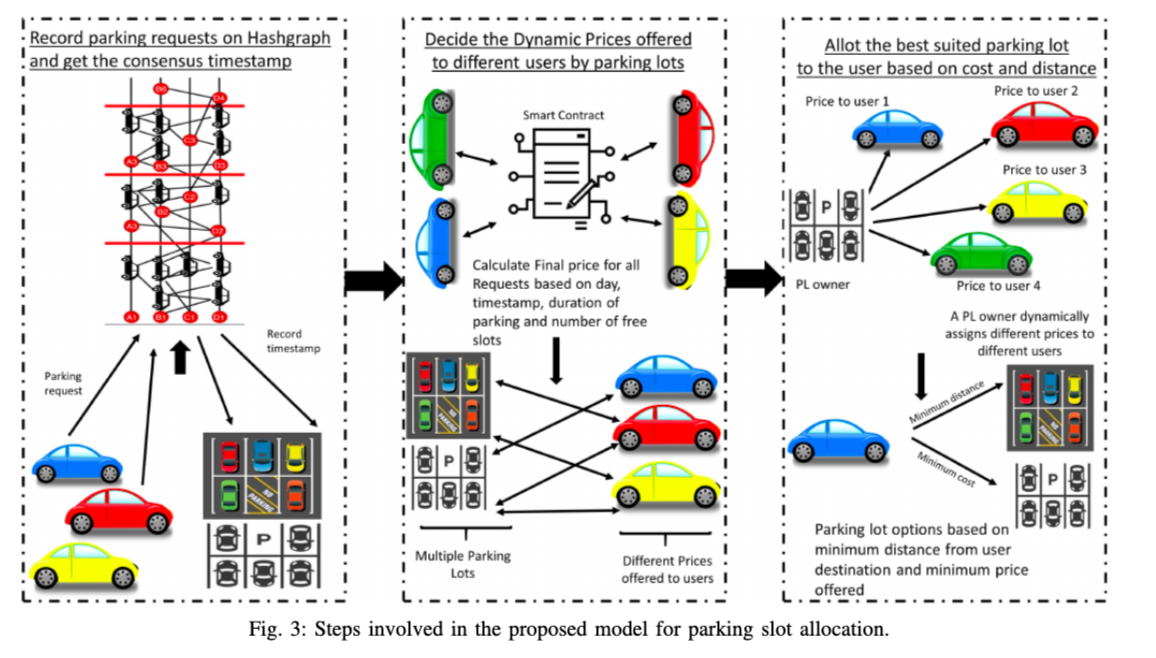